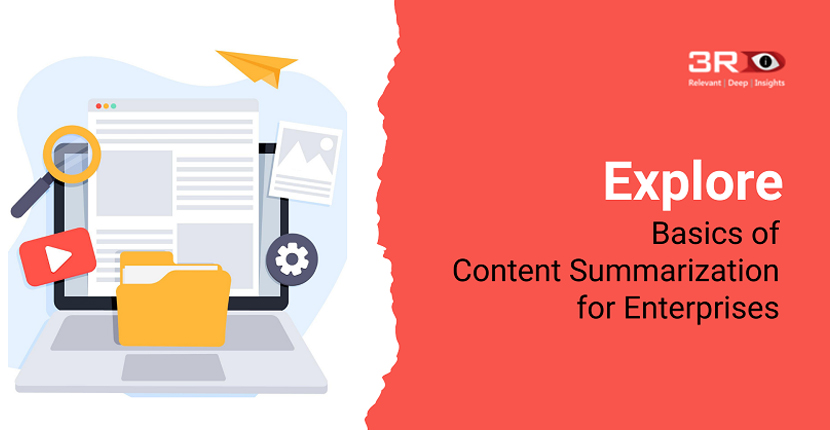
Basics of Content Summarization for Enterprises
Enterprise data often involves large volumes of data that is ever on the increase as more and more information keeps getting added from a plethora of different sources. In such a scenario, the need to analyze data in real time can become a challenge, as working with such large volumes of data is next to impossible. Also, there is repetitive data along with information that is of very little significance in the bigger scheme of things. An easy solution is to create a short summary of the essence of all that data and consider it for drawing relevant insights. This is where content summarization becomes useful as a functionality.
What is Content Summarization
The process of building a condensed and cohesive version of a longer document or large volumes of data is known as content summarization. It is the process of extracting the most significant data from a source of data in order to create a version of the same essence for a specific user or purpose.
Content summarization is a skill that most of us take for granted as the human brain is highly capable of deriving the key points in a certain amount of data or large volumes of content, and then use those points to create a coherent summary that still retains the information that was there in the original content. However, manually going through the large volumes of enterprise data, which keeps on increasing every minute, is next to impossible, which calls for the need of automation.
Benefits of Content Summarization
According to experts, the following are the key advantages of content summarization.
- Summaries help you save time by reducing the amount of time you spend reading.
- Summaries aid in the selection of documents when conducting research.
- The efficacy of indexing is improved by automatic summarization.
- Personalized information and personalized summaries are useful in question-answering systems.
- Using automated or partially automated summarization systems enables commercial content summarization services to increase the number of texts they are able to process.
Understanding the Approaches to Enterprise Content Summarization
When it comes to automated enterprise content summarization of enterprise data, there are 2 key approaches that are usually followed. These are as follows:
a] Extraction-based summarization
This technique of text summarization entails extracting essential words from a source document and combining them to create a summary. Without making any changes to the texts, the extraction is done according to the defined measure.
b] Abstraction-based summarization
Parts of the source document are paraphrased and shortened as part of the abstraction approach. When abstraction is used for text summarization in deep learning issues, it can overcome the extractive method's grammar errors.
The abstractive content summarization algorithms, similar to humans, create new phrases and sentences with the purpose of communicating the most important information from the original text. As a result, abstraction outperforms extraction.
The text summarizing algorithms required for abstraction, on the other hand, are more complex to build, which is why extraction is still widely used. We should expect advancements in this field as research progresses, allowing us to fluently and reliably shorten long text pages.
AI and Machine Learning for Content Summarization
Now that we've examined the importance of enterprise content summarization, the next step is to put it into practice. We have new age enterprise search platforms with content summarization features to help us with this. 3RDi Search is a good example of a content summarization platform that works well.
In addition to machine learning and semantics, the 3RDi Search content summarization platform is driven by AI and NLP technology. Before producing the requisite summary texts, machine learning models are frequently trained to comprehend documents and condense the useful information.
With so much data moving in the digital world, machine learning algorithms that can automatically condense lengthy texts and offer accurate summaries that carry the intended to deliver content summarization fluently are needed.
The content summarization algorithms required for abstraction, on the other hand, are more complex to build, which is why extraction is still widely used. We should expect advancements in this field as research progresses, allowing us to fluently and reliably shorten long text pages.
Want to explore the possibilities that a new age content summarization platform for effective content summarization? Visit www.3rdisearch.com/ or drop us an email on info@3rdisearch.com and our team will get in touch with you.
Read: Must Have Features of a Powerful Enterprise Search Engine